Researchers Find Limited Explanations in AI Might Benefit Consumers
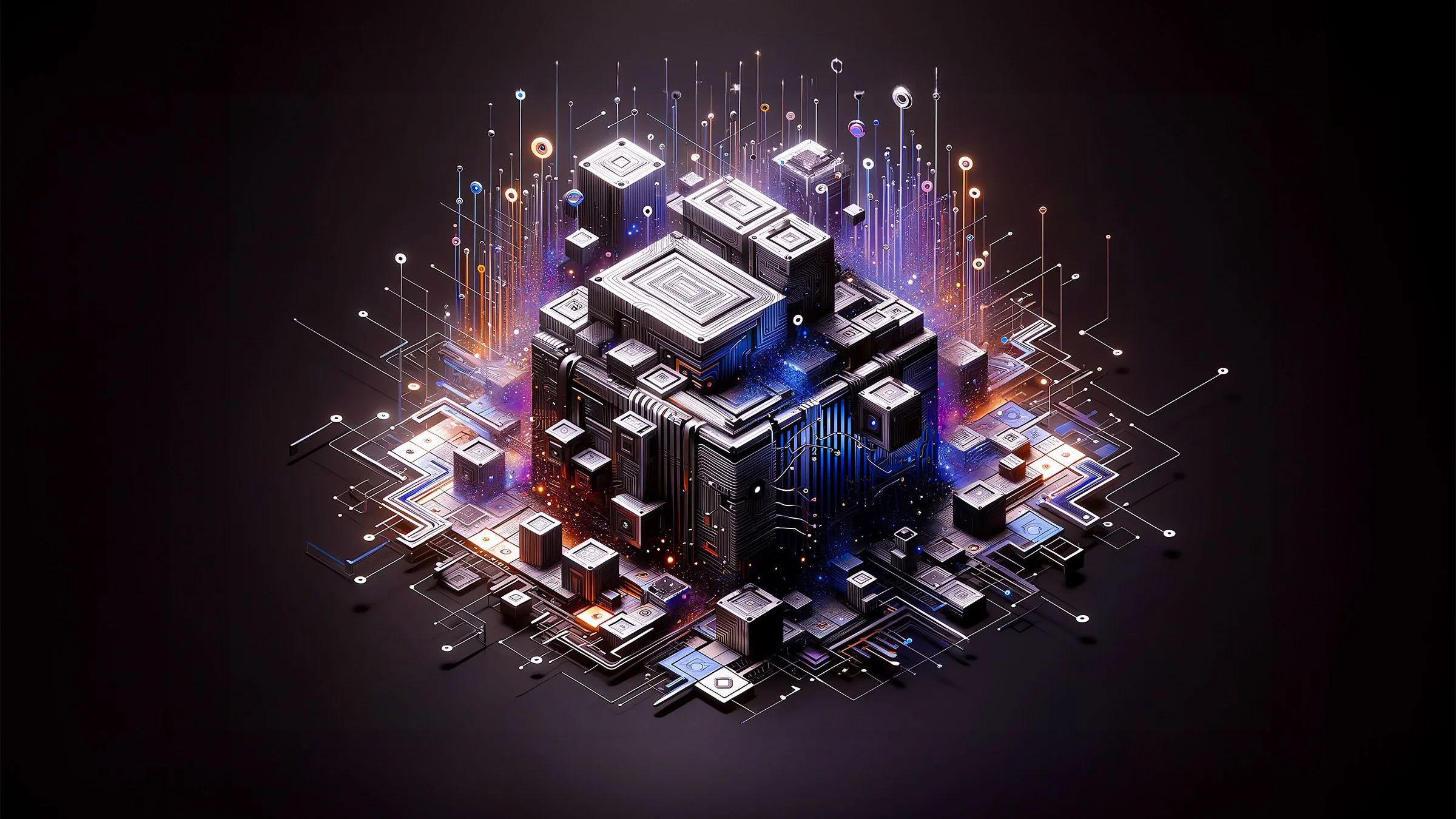
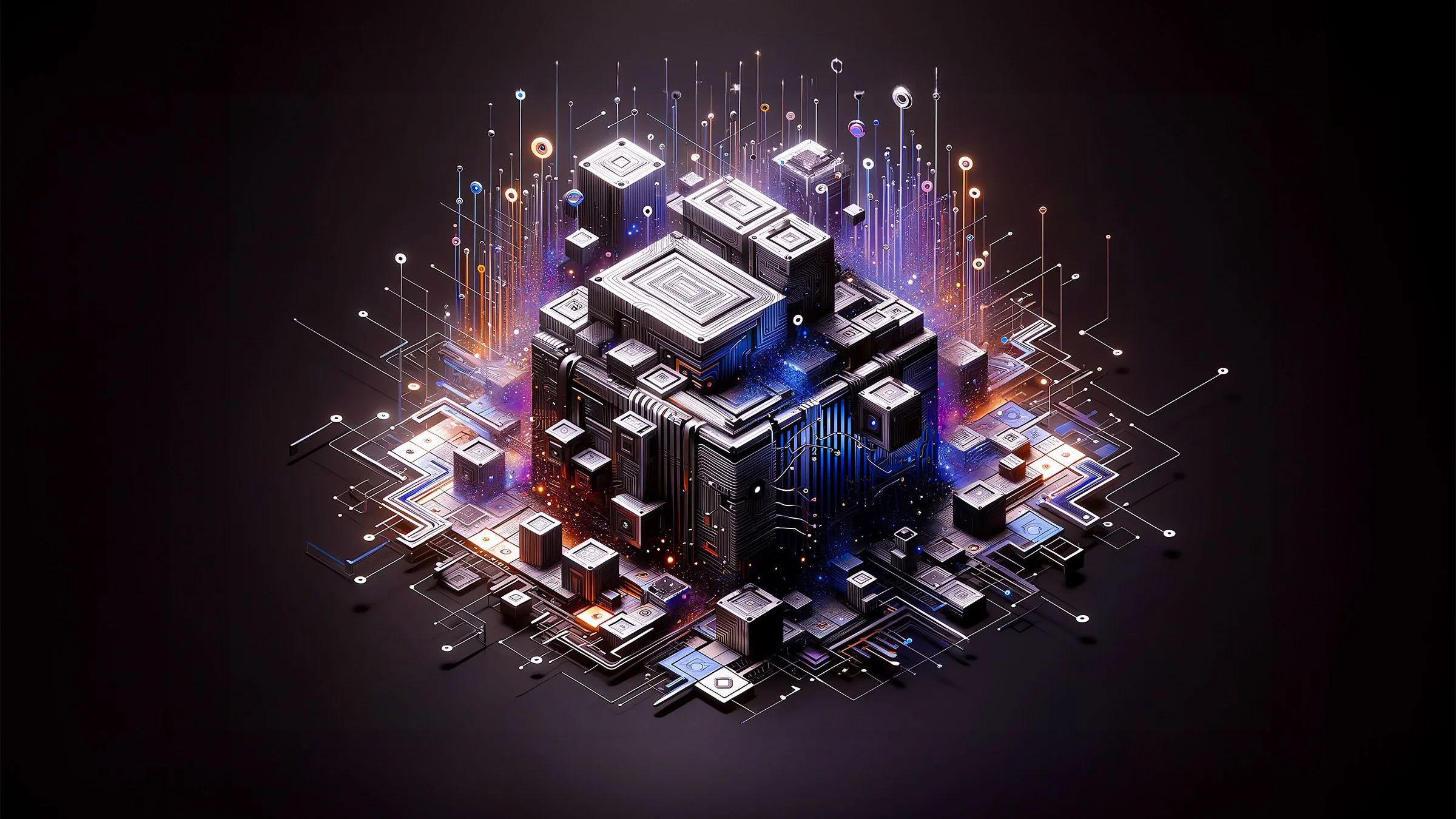
Academic Research by: Kannan Srinivasan
Recent algorithms in artificial intelligence (AI) are often referred to as “black box” models, meaning their inputs and operations are not visible to the user, making their decisions difficult to interpret. eXplainable AI (XAI) is a class of methods that seeks to address the lack of AI interpretability and trust by explaining AI decisions to customers.
Many experts believe that regulating AI by mandating fully transparent XAI leads to greater social welfare. However, a new study from the Tepper School and the University of Southern California found that such regulation may lead to less-than-optimal outcomes for both companies and consumers.
“Companies are facing pressure from legislators and customers to adhere to accountable AI practices, but we know little about the economic implications of XAI,” said Behnam Mohammadi, Ph.D. candidate at the Tepper School, who coauthored the study. “We took a deep dive into the complexities of XAI regulations to learn about their impact on competition among companies and social welfare.”
XAI aims to produce “glass box” models that are explainable while retaining prediction accuracy. Along with calls by consumer activists, XAI has been gaining traction across various industries.
The researchers studied a situation where two big companies dominate the market, specifically focusing on the insurance industry — a field that uses AI to decide rates. The study found that in places with no rules, companies and customers often want different levels of explanation from AI.
The study concluded that partial explanations might be better for both consumers and companies. Their key finding is that regulating an AI product to provide full explanations is not a recommended regulatory strategy. Instead, the optimal XAI policy would allow firms to offer flexible policies of optional XAI and to differentiate their XAI levels, which may aid social welfare.
“A one-size-fits-all policy across all markets, particularly one that mandates full explanation, may not yield the desired outcomes,” noted Tim Derdenger, associate professor of marketing and strategy at the Tepper School and study coauthor.
Kannan Srinivasan, study coauthor and professor of management and marketing at the Tepper School, said that as AI takes center stage in enterprises, a number of solutions are proposed to mitigate risks associated with AI. “Transparency is seen as a mechanism to alleviate potential bias in AI algorithms. Our analysis shows that may well not be the case,” said Srinivasan.